Workshop at 22.-24.09.2022
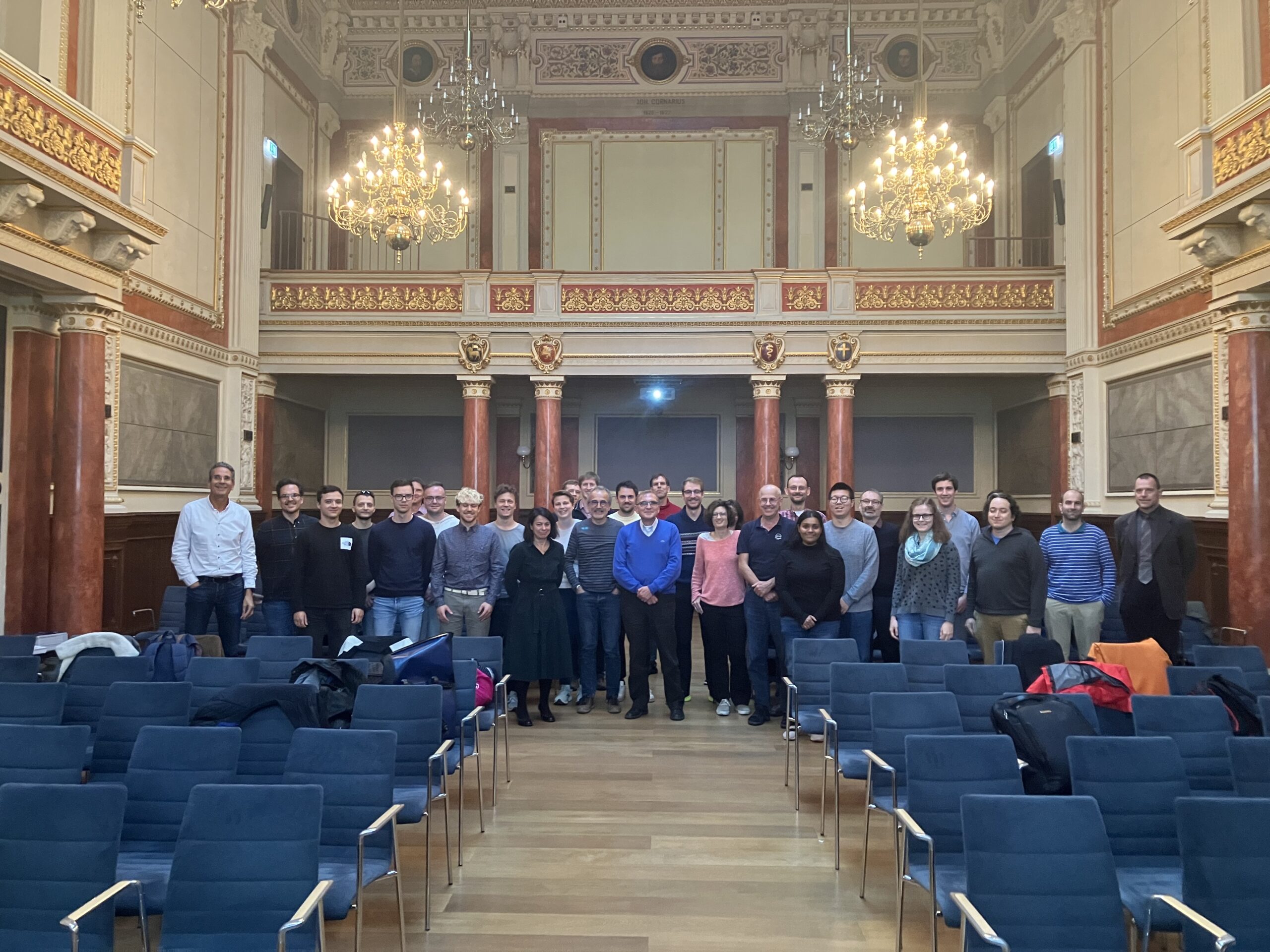
Speakers:
Yannick Baraud
Tugkan Batu
Quentin Bertet
Cristina Butucea
Alain Celisse
Alois Kneip
Victor Panaretos
Luc Pronzato
Richard Samworth
Martin Wahl
Yannick Baraud: Robust Estimation in Exponential Families
We observe a finite number of pairs of random variables that are presumed to be i.i.d. and we consider the problem of estimating the conditional distribution of the second coordinate given the first. We model this conditional distribution as an element of a given single-parameter exponential family for which the value of the parameter is an unknown function of the first coordinate of the pair. We provide an estimator of the conditional distribution based on our observations and analyse its performance not only when the statistical model is exact, as commonly done in statistics, but also when it is possibly misspecified (the pairs are independent but not exactly i.i.d., the true conditional distribution does not belong to the chosen exponential family but is only close to it, etc). We establish non-asymptotic risk bounds and show that our estimator is robust to a possible departure from the hypotheses we started from. Finally we provide an algorithm to compute the estimator in low or medium dimensions and compare its performance to that of the celebrated maximum likelihood estimator.
This is a joint work with Juntong Chen
Tugkan Batu: [Title to be announced]
Quentin Bertet: [Title to be announced]
Cristina Butucea: Off-the-grid estimation of sparse mixtures
We consider a general non-linear model where the signal is a finite mixture of an unknown, possibly increasing, number of features issued from a continuous dictionary parameterized by a real non-linear parameter. The signal is observed with Gaussian (possibly correlated) noise in either a continuous or a discrete setup.
We propose an off-the-grid optimization method to estimate both the non-linear parameters of the features and the linear parameters of the mixture.
We use recent results on the geometry of off-the-grid methods, to give minimal separation on the non-linear parameters such that interpolating certificate functions can be constructed. Using also tail bounds for suprema of Gaussian processes we bound the prediction risk with high probability. Our rates are up to log-factors similar to the rates attained by the Lasso predictor in the linear regression model. We also establish convergence rates that quantify with high probability the quality of estimation for both the linear and the non-linear parameters.
This is joint work with J.F. Delmas, A. Dutfoy and C. Hardy.
Alain Celisse: [Title to be announced]
Alois Kneip: [Title to be announced]
Victor Panaretos: [Title to be announced]
Luc Pronzato: Sequential online subsampling for thinning experimental designs
\(\def\ma{\alpha}\)We consider a parameter estimation problem where the design points \(X_i\) are i.i.d.\ with an unknown probability measure \(\mu\) and are presented sequentially. The objective is to select good candidates \(X_i\) on the fly when only a given proportion \(\ma\) of them can be selected, \(\ma\in(0, 1)\), in order to maximize a concave function \(\Phi\) of the information matrix.
The optimal solution corresponds to the construction of an optimal bounded design measure \(\xi_\ma^* \leq \mu/\ma\): the optimal acceptation rule is to select all \(X_i\) such that the directional derivative \(F_\Phi(\xi_\ma^* ,\delta_{X_i})\) of \(\Phi\) at \(\xi_\ma^* \) in the direction \(\delta_{X_i}\) is above the \((1-\ma)\)-quantile of that directional derivative in the direction \(\delta_X\), \(F_\Phi(\xi_\ma^* ,\delta_X)\), when \(X \sim\mu\). The difficulty is that \(\mu\) is unknown and \(\xi_\ma^*\) must be constructed online.
It is shown in [1] that selections based on the directional derivatives for the current design measure \(\xi_k\) (the empirical measure of design points already selected among the \(k\) presented so far) ensure convergence of that measure to the optimal one, \(\xi_\ma^*\). However, this requires estimating the \((1-\ma)\)-quantile of \(F_\Phi(\xi_k,\delta_X)\). It was suggested in [1] to estimate this quantile by using all \(X_i\)‘s already presented, but recursive estimation is possible [2], which yields a nonlinear two-time-scale stochastic approximation scheme. As only the current information matrix and estimated quantile
need to be stored, the construction can be applied to very long design sequences. Unlike IBOSS (Information-Based Optimal Subdata Selection) [3], it can be used on the fly and is not limited to a particular regression model and design criterion.
References:
[1] L. Pronzato. On the sequential construction of optimum bounded designs. Journal of Statistical Planning and
Inference, 136:2783-2804, 2006.
[2] L. Pronzato and H. Wang. Sequential online subsampling for thinning experimental designs. Journal of
Statistical Planning and Inference, 212:169-193, 2021.
[3] H. Wang, M. Yang, and J. Stufken. Information-based optimal subdata selection for big data linear regression.
Journal of the American Statistical Association, 114(525):393-405, 2019.
Richard Samworth: Optimal nonparametric testing of Missing Completely At Random, and its connections to compatibility
Given a set of incomplete observations, we study the nonparametric problem of testing whether data are Missing Completely At Random (MCAR). Our first contribution is to characterise precisely the set of alternatives that can be distinguished from the MCAR null hypothesis. This reveals interesting and novel links to the theory of Fréchet classes (in particular, compatible distributions) and linear programming, that allow us to propose MCAR tests that are consistent against all detectable alternatives. We define an incompatibility index as a natural measure of ease of detectability, establish its key properties, and show how it can be computed exactly in some cases and bounded in others. Moreover, we prove that our tests can attain the minimax separation rate according to this measure, up to logarithmic factors. Our methodology does not require any complete cases to be effective, and is available in the R package MCARtest.
Martin Wahl: Optimal estimation for linear SPDEs from multiple measurements
We consider the problem of parameter estimation for a second order linear stochastic partial differential equation (SPDE). Observing the solution to the SPDE continuously in time and averaged in space over a small window at multiple locations, we construct estimators for the diffusivity, transport and reaction coefficients, and show that their rates of convergence depend on the respective differential order (with the fastest rate achieved for the diffusivity coefficient and the slowest rate for the reaction terms). Moreover, we show that these rates are minimax-optimal by proving an explicit lower bound in the asymptotic regime where the number of measurements goes to infinity as the spatial window shrinks to zero. The proof of the minimax lower bounds relies on an explicit analysis of the reproducing kernel Hilbert space (RKHS) of the SPDE, and may be of independent interest. This is joint work with Randolf Altmeyer and Anton Tiepner.